Reinforced coloring for end-to-end instance segmentation
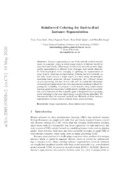
View/ Open
Date
2020-05-19Author
Tran, Anh Tuan
Nguyen, Tuan Khoa
Tran, Minh Quan
Jeong, Won-Ki
Metadata
Show full item recordAbstract
Instance segmentation is one of the actively studied research topics in computer vision in which many objects of interest should be separated individually. While many feed-forward networks produce high-quality segmentation on different types of images, their results often suffer from topological errors (merging or splitting) for segmentation of many objects, requiring post-processing. Existing iterative methods, on the other hand, extract a single object at a time using discriminative knowledge-based properties (shapes, boundaries, etc.) without relying on post-processing, but they do not scale well. To exploit the advantages of conventional single-object-per-step segmentation methods without impairing the scalability, we propose a novel iterative deep reinforcement learning agent that learns how to differentiate multiple objects in parallel. Our reward function for the trainable agent is designed to favor grouping pixels belonging to the same object using a graph coloring algorithm. We demonstrate that the proposed method can efficiently perform instance segmentation of many objects without heavy post-processing.
Collections
- Tran Minh Quan [10]