Dual consistency assisted multi-confident learning for the hepatic vessel segmentation using noisy labels
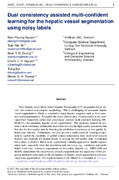
View/ Open
Date
2022Author
Nguyen, Phuong Nam
Vo, Van Tuan
Duong, T. M. Soan
Nguyen, D. Tr. Chanh
Bui, Trung
Truong, Q. H. Steven
Metadata
Show full item recordAbstract
Noisy hepatic vessel labels from Computer Tomography (CT) are popular due to vessels’ low-contrast and complex morphology. This is challenging for automatic hepatic vessel segmentation, which is essential to many hepatic surgeries such as liver resection and transplantation. To exploit the noisy labeled data, we proposed a novel semi-supervised framework called dual consistency assisted multi-confident learning (DC-Multi-CL) for automatic hepatic vessel segmentation. The proposed framework contains a dual consistency architecture that learns not only the high-quality annotation data but also the low-quality data by boosting the prediction consistency on low-quality labeled data robustly. Furthermore, we also present a multi-confident learning component to exploit the capability of global context information from multi-level network features and eradicate the human efforts on refining the low-quality data. Combining these ideas, we believe that it raises a potentially valuable approach to handle segmentation task, especially when the annotation data are noisy, e.g. unlabeled and mislabeled voxel-wise. Extensive experiments on two public datasets, i.e. 3DIRCADb and MSD8, demonstrate the effectiveness of each component and the superiority of the proposed method to other state-of-the-art methods in hepatic vessel segmentation and semi-supervised segmentation. The implementation of DC-Multi-CL is available at: https://github.com/VinBrainJSC/DualConsistency_Mutil-CL.git.