On the trade-off between privacy protection and data utility for chest X-ray images
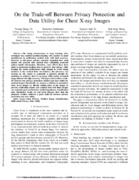
Năm xuất bản
2022Tác giả
Vu, Truong Giang
Wong, Kok-Seng
Nguyen, Anh Tu
Makhanov, Nursultan
Metadata
Hiển thị đầy đủ biểu ghiTóm tắt
Abstract
The advancement of deep learning (DL) techniques has significantly enhanced the accuracy of machine learning (ML) models in medical tasks. However, this progress raises privacy concerns, particularly regarding the handling of sensitive patient health information. Efforts have been made to anonymize medical data to protect privacy while maintaining high model performance. Despite these efforts, adversaries could potentially develop ML models capable of re-identifying patients by matching chest X-ray images with public or leaked datasets. This paper explores the balance between privacy protection and data utility for medical images. We propose a method to anonymize chest X-ray images by introducing noise directly to the images, aimed at preventing verification attacks. Our approach is evaluated for its effectiveness in preserving data utility for lung disease classification. Simulation results on real-world datasets demonstrate that our method achieves a favorable trade-off between privacy protection and classification performance.
Định danh
https://vinspace.edu.vn/handle/VIN/239Collections
- Kok-Seng Wong, PhD [11]